6Event Correlation Approaches and Techniques
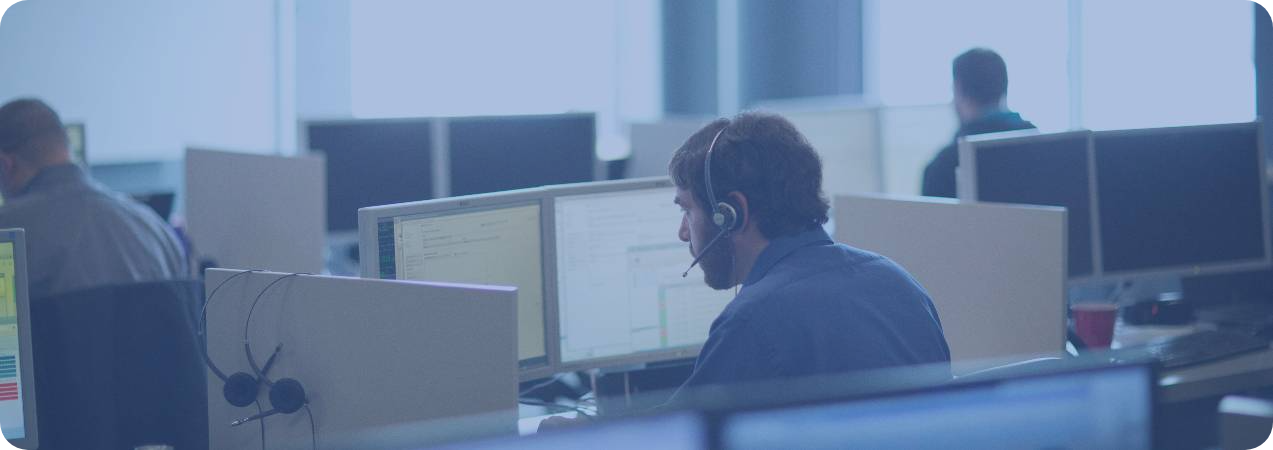
There are multiple ways to identify relationships in event data and determine causation. Here's a look at the most common, all of which we perform.
Time-based event correlation
This technique identifies relationships between events based on when they occurred. For example, if a router goes down shortly after a configuration change, time-based correlation would flag the potential relationship. In one client environment, we used time-based correlation to identify that a recurring network issue was happening precisely when a scheduled database backup job ran. This wasn't obvious from looking at individual alerts, but the correlation pattern made it clear.
Rule-based event correlation
While traditional rule-based approaches require manual rule creation for each scenario, our platform combines predefined rules with machine learning to adapt to new patterns automatically. We might initially create a rule that correlates database timeouts with high CPU utilization on the database server. Over time, our system learns additional patterns, such as correlating those same timeouts with network congestion during peak hours, without requiring manual rule updates.
Pattern-based event correlation
Our system recognizes common failure patterns across client environments. As it encounters new patterns, machine learning algorithms incorporate them into the knowledge base. One client experienced a unique failure pattern where a specific type of network switch would generate a sequence of seemingly unrelated alerts before failing completely. After observing this pattern twice, our system was able to automatically identify the early warning signs and predict the impending failure before it happened again.
Topology-based event correlation
By maintaining an accurate CMDB with detailed topology information, our platform can trace the relationships between components. When an issue occurs, the system can map affected nodes and identify the most likely source of the problem. For a financial services client with a complex multi-tier application architecture, topology-based correlation was crucial. When users reported application slowness, our system could trace the dependencies from the web servers to application servers to databases, identifying a storage bottleneck as the root cause rather than focusing on the front-end symptoms.
Domain-based event correlation
Our correlation engine works across multiple domains, including network performance, application performance, and infrastructure health, providing a comprehensive view of the environment. In one case, we were able to correlate application timeout errors (application domain) with network congestion (network domain) and storage latency (infrastructure domain) to identify a holistic problem that crossed traditional IT silos.
History-based event correlation
The platform learns from historical events, recognizing similarities between current issues and past incidents to suggest proven resolution steps. For example, after resolving several incidents related to a specific type of database error, our system now automatically suggests the most effective troubleshooting steps based on what worked in previous similar situations.
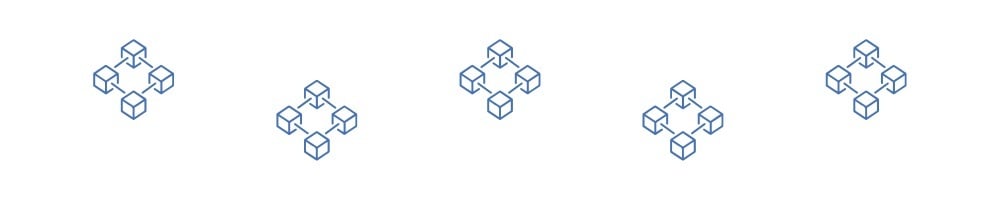